|
Will give their core data to others. train. In addition, how to reasonably label and annotate the data is also very important. Data classification can help products improve efficiency, while high-precision annotation data can further enhance the professional performance of large models. However, at this stage, the cost for vertical industries to obtain high-precision annotation data is relatively high, and there is less industry-specific data in public databases. Therefore, high requirements are placed on the construction of large vertical models. Generally speaking, if you want to build a large vertical model, the importance of data far exceeds computing power and algorithms.
Data has become a "stuck point" for enterprises to break through large vertical models. 3. Stay one step ahead with industry data. Vertical large-scale models emphasize application and scenario-first logic, and in China, the value Argentina WhatsApp Number of the industry side is even more emphasized. On the one hand, under the current wave of intelligence in China, there is a broad market demand for digital innovation on the industrial side; on the other hand, under the toB ecosystem,
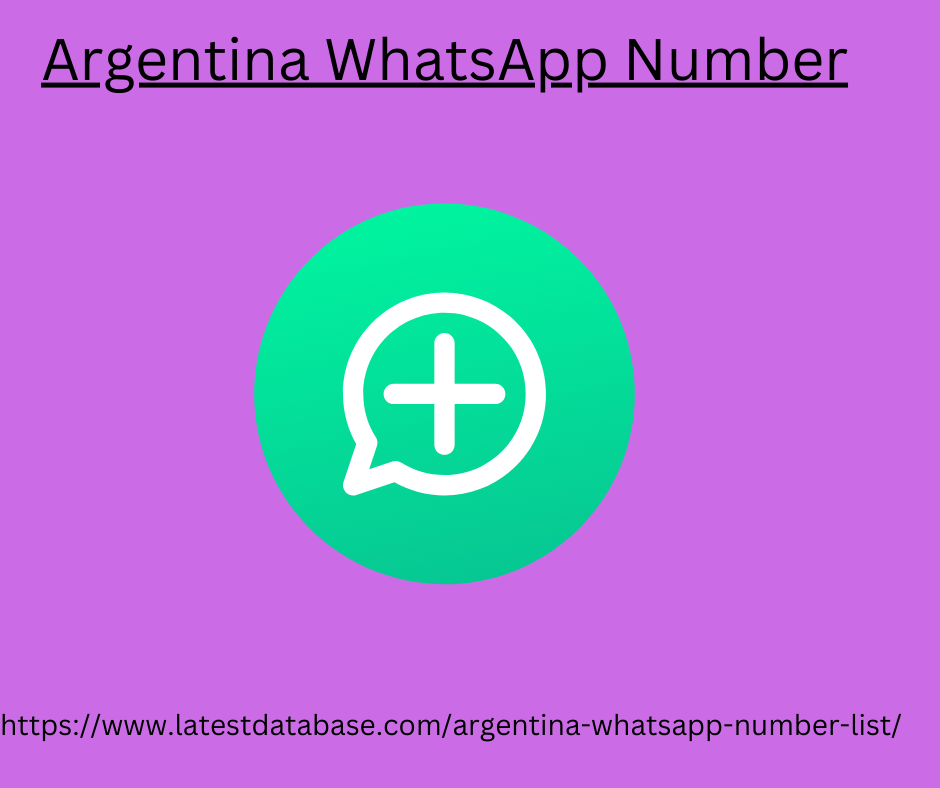
practices based on vertical applications are also conducive to the formation of data flywheels and scene flywheels. The premise of all this is that companies that launch large-scale vertical models have established technical barriers and moats in the industry, that is, the competitive advantage of "no one has what I have". From this point of view, companies that have been deeply involved in vertical industries for many years may have a greater chance of winning. This type of enterprise has a relatively deep accumulation in data level, large models and knowledge graphs,
|
|